SPECT is a commonly used approach to clinical evaluation of coronary artery disease. It visualizes functional information of the Left Ventricle (LV) in 3D, such as discontinuous perfusion scintigrams due to scarred or ischemic myocardium. Gated cardiac SPECT also provides cardiac motion information along the time dimension. The segmentation of LV epicardial and endocardial borders allows quantitative analysis of perfusion defects and cardiac function, and hence is of significant importance to diagnosis and clinical study. In SPECT, normal perfusion has high brightness in good contrast against the dark background, while perfusion defects have low or no brightness and hence low or no contrast against the background. Moreover, SPECT imaging can suffer from motion artefacts due to long imaging times, which is not a trivial task to compensate.
Accurate determination of the LV borders around the defect is difficult as there are few or no defined image features, such as gradients. Additionally, the image data very often contains hot structures in the proximity of the LV, such as the liver, considered as perfusion artifacts that impose similar difficulties for correct LV segmentation. Furthermore, although resolution recovery techniques can be applied to increase SPECT spatial resolution, SPECT images have far lower resolution, as well as exhibit fewer anatomical landmarks (since the images suffer degradation due to various types of attenuation), in comparison to other modalities, such as CT and MRI.
Beyond analysis in static LV data, segmentation of LV in 4D allows analysis of cardiac motion. In this work, we propose a novel spatiotemporal constraint based on shape and appearance and combine it with a level set deformable model for LV segmentation in 4D gated cardiac SPECT, particularly in the presence of perfusion defects. The model incorporates appearance and shape information into a soft-to-hard probabilistic constraint, and utilizes spatiotemporal regularization via a Maximum A Posteriori (MAP) framework. This constraint force allows more lexibility than the rigid forces of shape constraint-only schemes, as well as other state of the art joint shape and appearance constraints. The combined model can hypothesize defective LV borders based on prior knowledge. We present comparative results to illustrate the improvement gain. A brief defect detection example is finally presented as an application of the proposed method.
Proposed CCACE Model
The proposed model comprises two stages: training and segmentation. Through
the training stage, sets of pixel-wise Gaussian and spatiotemporal priors are obtained. The Gaussian priors include a prior image, a prior shape, an image variation term, a shape variation term, and the correlation between image and shape. The spatiotemporal priors are the products of applying PCA to global-to-local transformation parameters of the shape variations, and include mean of the
parameters, eigenmodes (i.e. modes of variations), weights for the eigenmodes, as well as the covariance of the weights.
During segmentation, an initial surface embedded in level sets is placed in the input image. A constraint force is derived by finding the maximum of the multivariate pdf of the input image and shape (the evolving level sets) based on the Gaussian priors from the training stage. As the level sets evolve, the pdf continuously updates by aligning the prior image and shape with the input image and shape via global-to-local transformations to enable meaningful evaluation of the pdf. The alignment is regularized by the prior distribution over a set of spatiotemporal parameters of the transformations. The force derived from this regularized multivariate pdf, effectively a posterior, is a shape and appearance-based spatiotemporal constraint obtained via MAP estimation. The evolving level sets update according to the combination of CACE forces and this constraint to reach (and hypothesize missing or defective) LV borders.
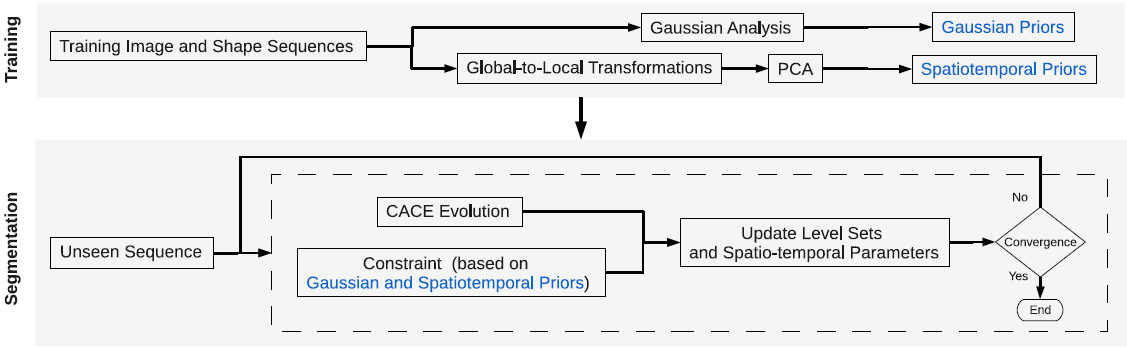 |
The proposed CCACE model. |
Example results - mid-slices containing perfusion defects and artefacts
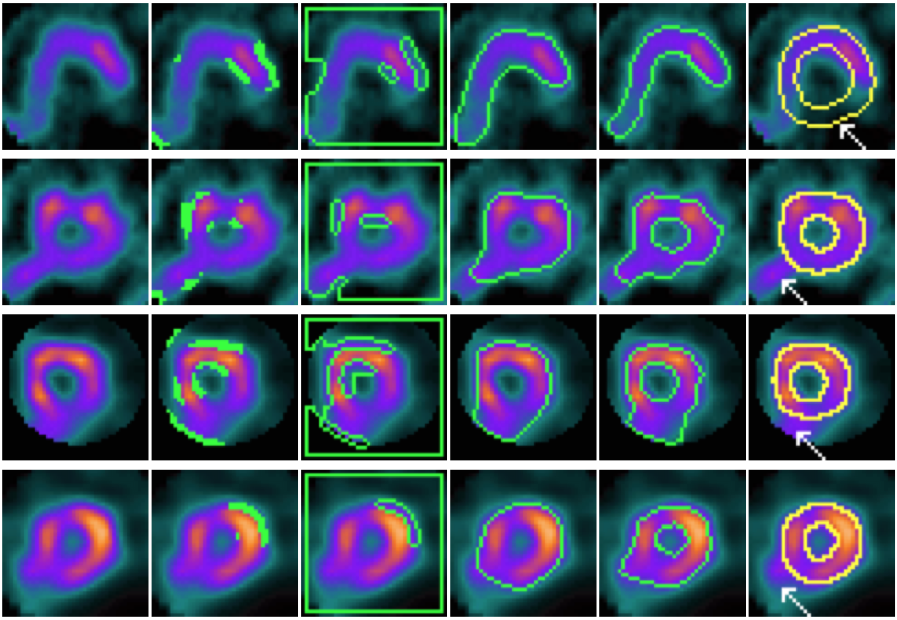 |
CACE and other models on mid-slices containing perfusion defects and artefacts (marked by white arrows). From left to right: input slice, results of CPM, geodesic snake, GVF geodesic snake, CACE, and the ground truth. |
Example results - gated cardiac SPECT with artifacts and severe perfusion defects
SCMS | 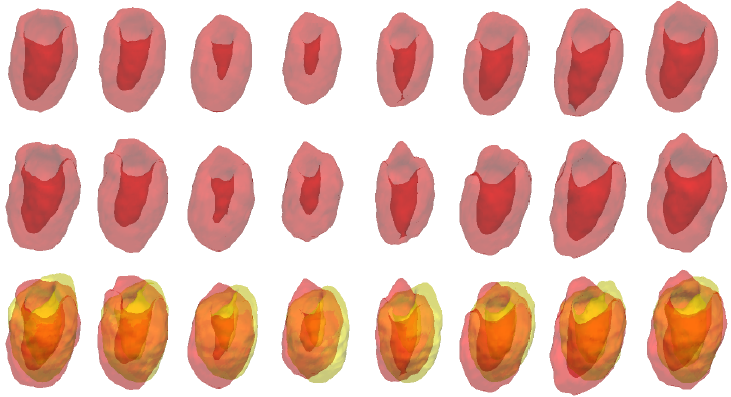 |
SCGAR | 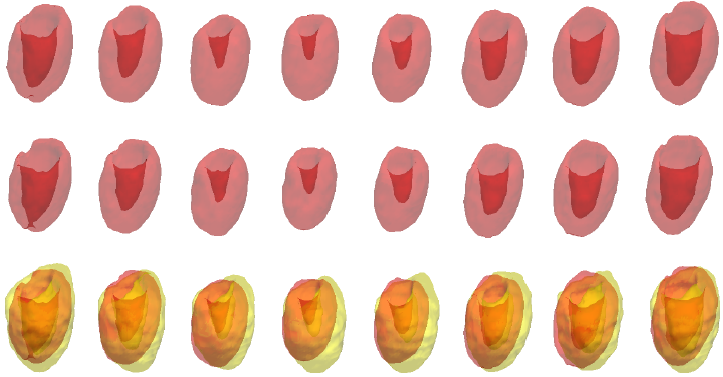 |
CCACE | 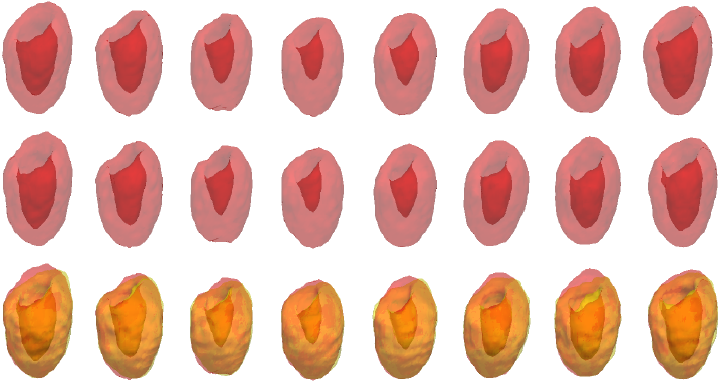 |
| Iterations of SCMS, SCGAR and CCACE on gated cardiac SPECT with artifacts and severe perfusion defects. Final row of each model shows the results overlaid on the ground truth. |
Example results - a large LV with long apical region
SCMS | 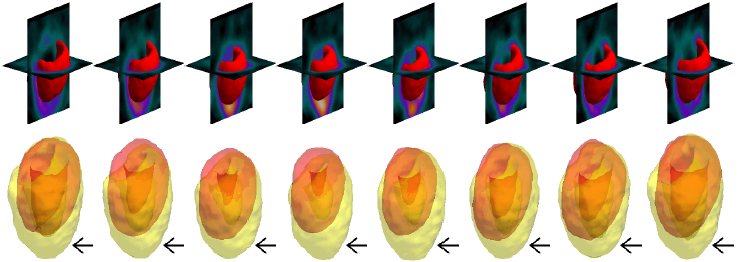 |
SCGAR | 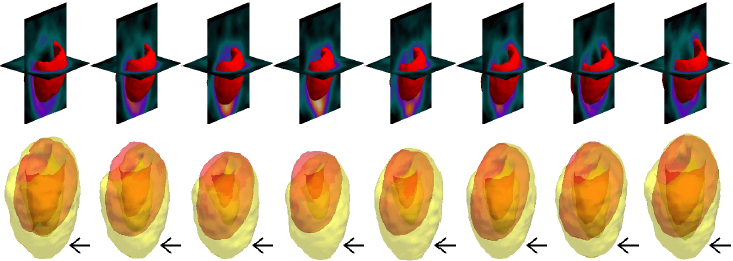 |
CCACE | 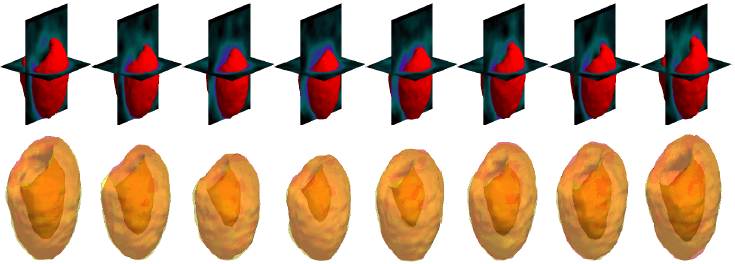 |
| Models' results on a large LV with long apical region. Black arrows indicate significant areas of mismatch against the ground truth. |
Example results - selected slices within middle region of the LV
SCMS | 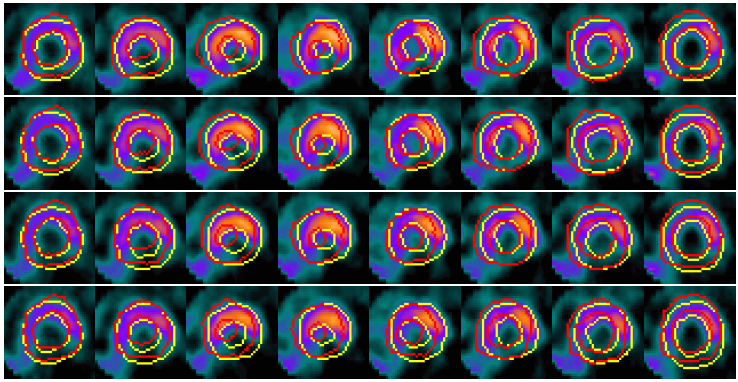 |
SCGAR | 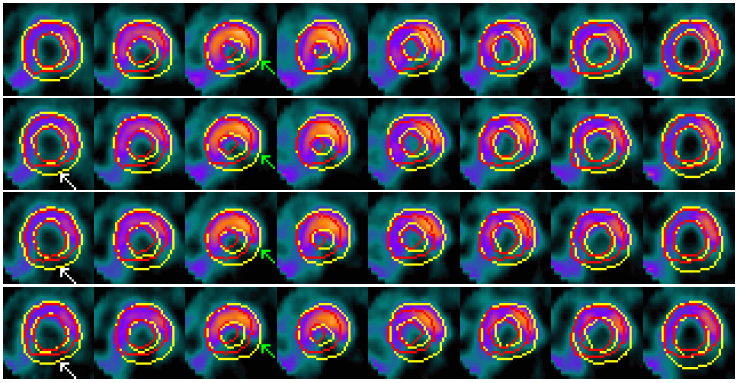 |
CCACE | 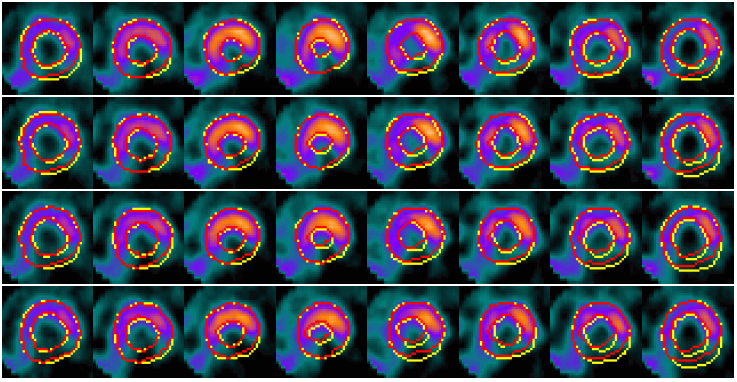 |
| Models' results on LV slices selected from the sequence. From top to bottom in each group: four slices at different locations within the middle region of the LV. |
Example results - selected slices within apical region of the LV
SCMS | 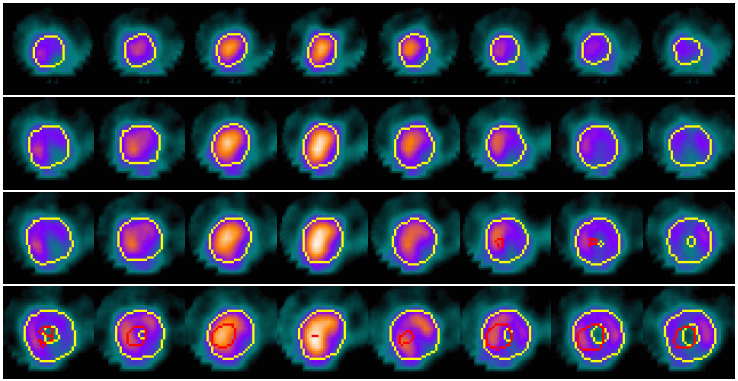 |
SCGAR | 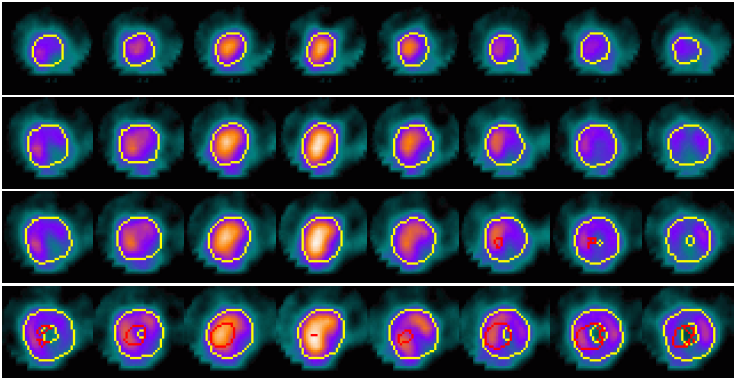 |
CCACE | 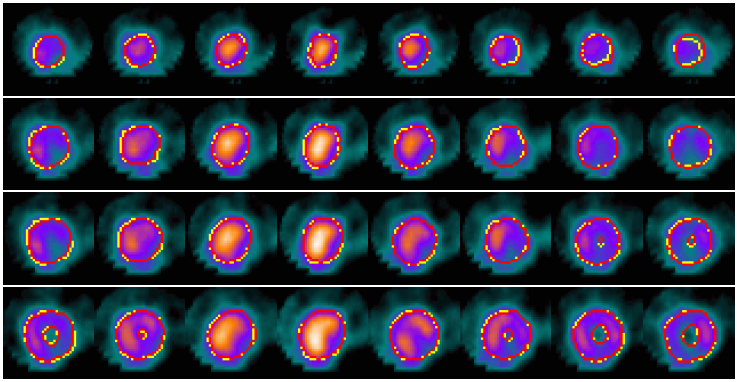 |
| Models' results on LV slices selected from the sequence. From top to bottom in each group: four slices at different locations within the apical region of the LV. |
Example results - defect detection in 2D slices
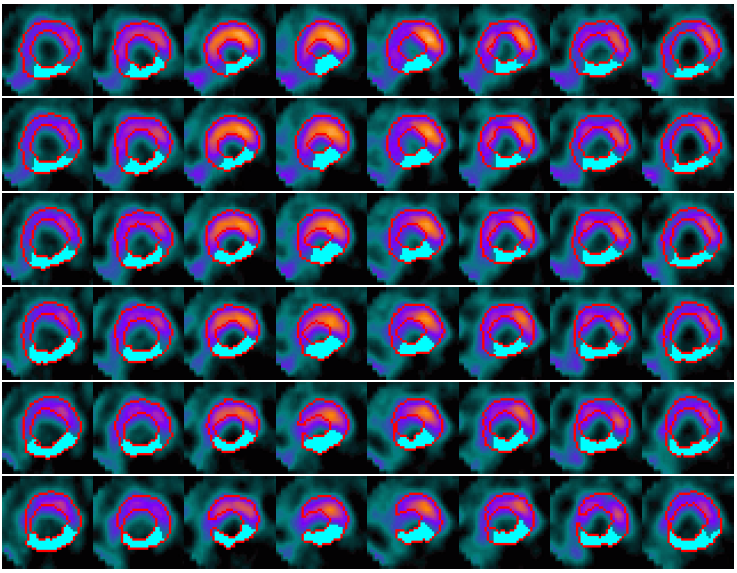 |
Defect detection in 2D slices by applying CACE to the segmented image based on the CCACE result. |
Example results - defect detection - more
 |
Automated defect detection by applying CACE to the segmented image based on the CCACE result. |
Publications
- R. Yang, M. Mirmehdi, X. Xie, and D. Hall, Shape and Appearance Priors for Level Set-based LV Segmentation, IET Journal of Computer Vision, vol. 7, no.3, pp. 170-183, 2013.
- Ronghua Yang, Majid Mirmehdi, Xianghua Xie and David Hall, Shape and Appearance based Spatiotemporal Constraint for LV Segmentation in 4D Cardiac SPECT, In Proceedings of Cardiovascular Interventional Imaging and Biophysical Modelling, A MICCAI Workshop, September 2009.